Real-time collaboration for Jupyter Notebooks, Linux Terminals, LaTeX, VS Code, R IDE, and more,
all in one place. Commercial Alternative to JupyterHub.
Real-time collaboration for Jupyter Notebooks, Linux Terminals, LaTeX, VS Code, R IDE, and more,
all in one place. Commercial Alternative to JupyterHub.
Path: blob/main/09. Machine Learning with Python/04. Clustering/03. Density-based Clustering.ipynb
Views: 4598
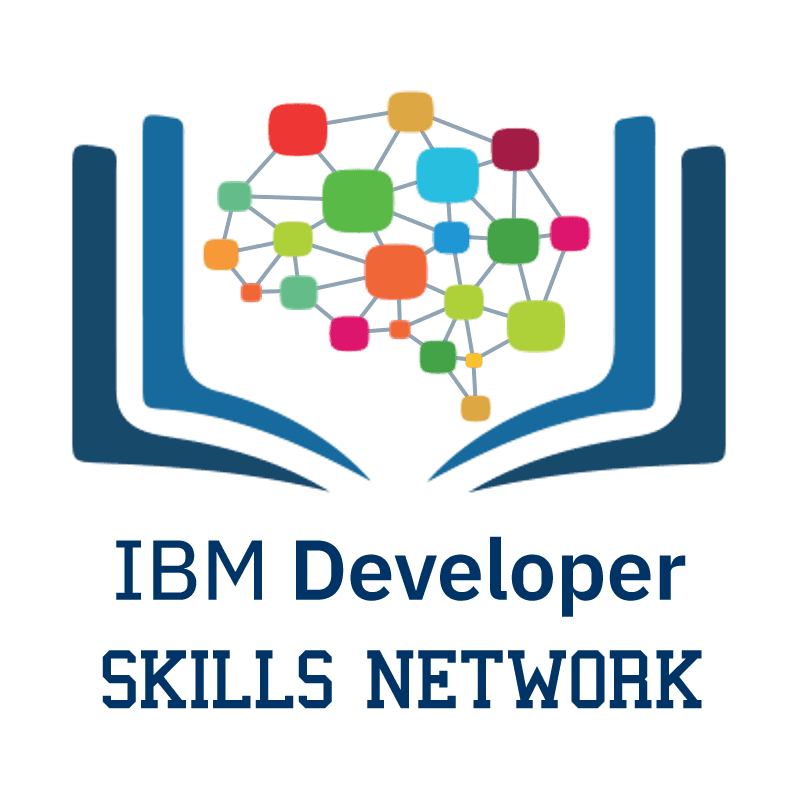
Density-Based Clustering
Objectives
After completing this lab you will be able to:
Use DBSCAN to do Density based clustering
Use Matplotlib to plot clusters
Most of the traditional clustering techniques, such as k-means, hierarchical and fuzzy clustering, can be used to group data without supervision.
However, when applied to tasks with arbitrary shape clusters, or clusters within a cluster, the traditional techniques might be unable to achieve good results. That is, elements in the same cluster might not share enough similarity or the performance may be poor. Additionally, Density-based clustering locates regions of high density that are separated from one another by regions of low density. Density, in this context, is defined as the number of points within a specified radius.
In this section, the main focus will be manipulating the data and properties of DBSCAN and observing the resulting clustering.
Import the following libraries:
- numpy as np
- DBSCAN from sklearn.cluster
- make_blobs from sklearn.datasets
- StandardScaler from sklearn.preprocessing
- matplotlib.pyplot as plt
Remember %matplotlib inline to display plots
Data generation
The function below will generate the data points and requires these inputs:
- centroidLocation: Coordinates of the centroids that will generate the random data.
- Example: input: [[4,3], [2,-1], [-1,4]]
- numSamples: The number of data points we want generated, split over the number of centroids (# of centroids defined in centroidLocation)
- Example: 1500
- clusterDeviation: The standard deviation of the clusters. The larger the number, the further the spacing of the data points within the clusters.
- Example: 0.5
Use createDataPoints with the 3 inputs and store the output into variables X and y.
Modelling
DBSCAN stands for Density-Based Spatial Clustering of Applications with Noise. This technique is one of the most common clustering algorithms which works based on density of object. The whole idea is that if a particular point belongs to a cluster, it should be near to lots of other points in that cluster.
It works based on two parameters: Epsilon and Minimum Points Epsilon determine a specified radius that if includes enough number of points within, we call it dense area minimumSamples determine the minimum number of data points we want in a neighbourhood to define a cluster.
Distinguish outliers
Let's Replace all elements with 'True' in core_samples_mask that are in the cluster, 'False' if the points are outliers.
Data visualization
Practice
To better understand differences between partitional and density-based clustering, try to cluster the above dataset into 3 clusters using k-Means. Notice: do not generate data again, use the same dataset as above.
Weather Station Clustering using DBSCAN & scikit-learn
DBSCAN is especially very good for tasks like class identification in a spatial context. The wonderful attribute of DBSCAN algorithm is that it can find out any arbitrary shape cluster without getting affected by noise. For example, this following example cluster the location of weather stations in Canada. <Click 1> DBSCAN can be used here, for instance, to find the group of stations which show the same weather condition. As you can see, it not only finds different arbitrary shaped clusters, can find the denser part of data-centred samples by ignoring less-dense areas or noises.
Let's start playing with the data. We will be working according to the following workflow:
Loading data
Overview data
Data cleaning
Data selection
Clustering
About the dataset
Environment Canada Monthly Values for July - 2015
[removed] [removed] table { font-family: arial, sans-serif; border-collapse: collapse; width: 100%; }td, th { border: 1px solid #dddddd; text-align: left; padding: 8px; }
tr:nth-child(even) { background-color: #dddddd; }
Name in the table | Meaning |
---|---|
Stn_Name | Station Name</font |
Lat | Latitude (North+, degrees) |
Long | Longitude (West - , degrees) |
Prov | Province |
Tm | Mean Temperature (°C) |
DwTm | Days without Valid Mean Temperature |
D | Mean Temperature difference from Normal (1981-2010) (°C) |
Tx | Highest Monthly Maximum Temperature (°C) |
DwTx | Days without Valid Maximum Temperature |
Tn | Lowest Monthly Minimum Temperature (°C) |
DwTn | Days without Valid Minimum Temperature |
S | Snowfall (cm) |
DwS | Days without Valid Snowfall |
S%N | Percent of Normal (1981-2010) Snowfall |
P | Total Precipitation (mm) |
DwP | Days without Valid Precipitation |
P%N | Percent of Normal (1981-2010) Precipitation |
S_G | Snow on the ground at the end of the month (cm) |
Pd | Number of days with Precipitation 1.0 mm or more |
BS | Bright Sunshine (hours) |
DwBS | Days without Valid Bright Sunshine |
BS% | Percent of Normal (1981-2010) Bright Sunshine |
HDD | Degree Days below 18 °C |
CDD | Degree Days above 18 °C |
Stn_No | Climate station identifier (first 3 digits indicate drainage basin, last 4 characters are for sorting alphabetically). |
NA | Not Available |
1- Load the dataset
We will import the .csv then we creates the columns for year, month and day.
2-Cleaning
Let's remove rows that don't have any value in the Tm field.
3-Visualization
Visualization of stations on map using basemap package. The matplotlib basemap toolkit is a library for plotting 2D data on maps in Python. Basemap does not do any plotting on it’s own, but provides the facilities to transform coordinates to a map projections.
Please notice that the size of each data points represents the average of maximum temperature for each station in a year.
4- Clustering of stations based on their location i.e. Lat & Lon
DBSCAN form sklearn library can run DBSCAN clustering from vector array or distance matrix. In our case, we pass it the Numpy array Clus_dataSet to find core samples of high density and expands clusters from them.
As you can see for outliers, the cluster label is -1
5- Visualization of clusters based on location
Now, we can visualize the clusters using basemap:
6- Clustering of stations based on their location, mean, max, and min Temperature
In this section we re-run DBSCAN, but this time on a 5-dimensional dataset: