Real-time collaboration for Jupyter Notebooks, Linux Terminals, LaTeX, VS Code, R IDE, and more,
all in one place. Commercial Alternative to JupyterHub.
Real-time collaboration for Jupyter Notebooks, Linux Terminals, LaTeX, VS Code, R IDE, and more,
all in one place. Commercial Alternative to JupyterHub.
Path: blob/main/diffusers_doc/en/img2img.ipynb
Views: 2542
Text-guided image-to-image generation
The StableDiffusionImg2ImgPipeline lets you pass a text prompt and an initial image to condition the generation of new images.
Before you begin, make sure you have all the necessary libraries installed:
Get started by creating a StableDiffusionImg2ImgPipeline with a pretrained Stable Diffusion model like nitrosocke/Ghibli-Diffusion
.
Download and preprocess an initial image so you can pass it to the pipeline:
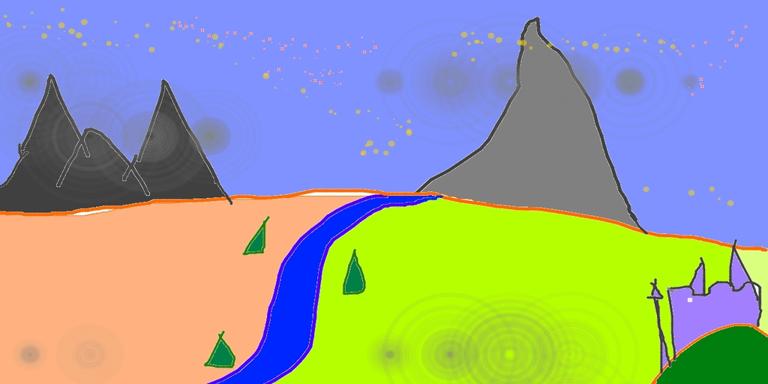
💡 strength
is a value between 0.0 and 1.0 that controls the amount of noise added to the input image. Values that approach 1.0 allow for lots of variations but will also produce images that are not semantically consistent with the input.
Define the prompt (for this checkpoint finetuned on Ghibli-style art, you need to prefix the prompt with the ghibli style
tokens) and run the pipeline:
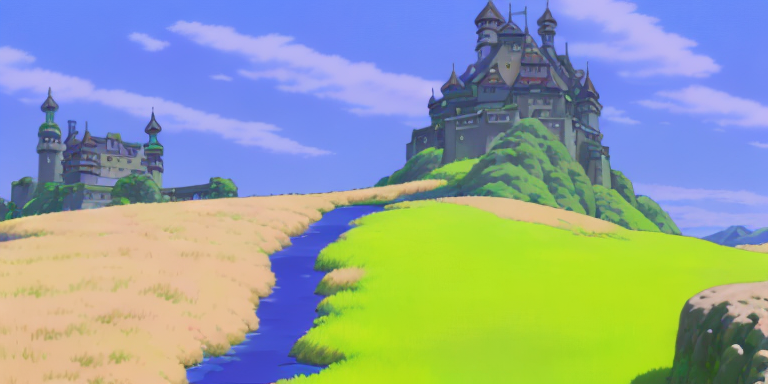
You can also try experimenting with a different scheduler to see how that affects the output:
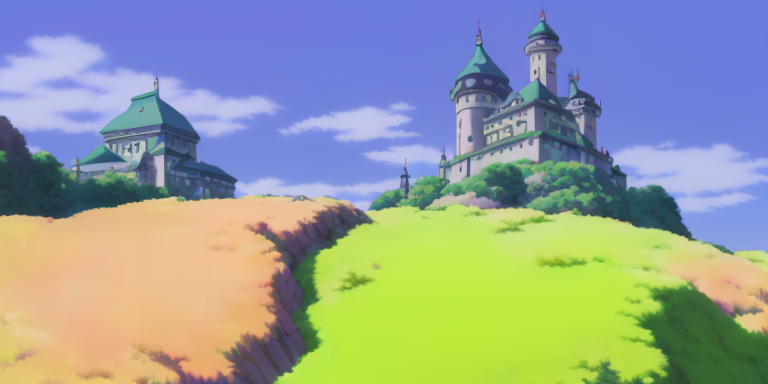
Check out the Spaces below, and try generating images with different values for strength
. You'll notice that using lower values for strength
produces images that are more similar to the original image.
Feel free to also switch the scheduler to the LMSDiscreteScheduler and see how that affects the output.