Real-time collaboration for Jupyter Notebooks, Linux Terminals, LaTeX, VS Code, R IDE, and more,
all in one place. Commercial Alternative to JupyterHub.
Real-time collaboration for Jupyter Notebooks, Linux Terminals, LaTeX, VS Code, R IDE, and more,
all in one place. Commercial Alternative to JupyterHub.
4. Graphing
Graphing your data shows relationships more clearly than presenting the same information in a table. A picture is worth a thousand words, so a well-made graph can save a lot of writing when you report your results.
4.1 Rough Graphs
Rough graphs are made for your own benefit. They don't need to be as polished as graphs that will appear in a report, so they can hand-drawn. Graphing each point as you take it is not a good idea, because that is inefficient. It can prejudice you about the value of the next data point. So take a set data points and then graph them all.
It is very useful to make rough graphs during lab when you have the chance to do something about any problems. You'll know if you need to check any data point, since any strange measurement will stand out in a graph. You'll also be able to spot regions where you should take more data. Typically, people take approximately evenly spaced data points over the entire range of the controllable (or “independent”) variable, which is certainly a good way to start. A graph of that inital data will tell you if there are regions where you should look more closely: regions where your graph is changing rapidly, going through a minimum or maximum, or changing curvature, for example. The graph helps you identify interesting sections where you should get more data, and saves you from taking lots of data in regions where little is happening.
For example, Figure 4.1 below shows the initial data taken for a phenomenon called mechanical resonance. All you need to know about resonance is that the amplitude (a measure of the response of an oscillating system) depends on the frequency of oscillating force driving the system. Notice that the driving frequencies were approximately evenly spaced. For the apparatus used, the highest and lowest frequencies attainable were easy to find, and the experimenter chose to space the frequencies evenly to get roughly 10 different frequencies between the extremes. From the graph of the initial data, you can see that the response doesn't change very much at either very high or very low frequencies, but something interesting happens near 5 Hz.

Figure 4.1: Initial set of data (filled circles) for amplitude response versus driving frequency for a resonant system. Notice that the data points are evenly spaced.
Suppose that the experimenter noticed this, too, and went back to take more data in the interesting range of frequencies. The frequency spacing used the second time is smaller than used in the first set by about a factor of ten, yielding 15 more measurements in the interest region. The result of adding the second set of measurements is shown in Figure 4.2. As you can see, the shape of the graph is now much better defined. Also, the additional data show that the high amplitude at 4.5 Hz was not due to a mistake. The experimenter could, of course, have taken data with the closer spacing over the entire frequency range, but that would waste time on measurements at both low and high frequencies where nothing much is happening. The strategy of taking coarsely spaced data and then backing up to take more data in interesting regions is a good compromise between completeness and efficiency, but you usually can’t identify the interesting regions without making a graph.
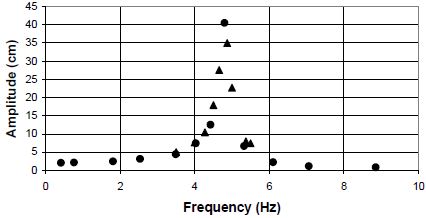
Figure 4.2: Amplitude vs. driving frequency in a resonant system, after adding more data points (triangles) around 4 to 6 Hz.
4.2 Polished Graphs
For reports, you should produce more polished graphs using a computer.
In particular, data points plotted on any graph should include uncertainty bars (sometimes called error bars) showing the uncertainty range associated with each data point. You should show both vertical and horizontal uncertainty bars, if they are both known. If the uncertainties are too small to be visible on the graph, you should mention that in your report so it's clear that you haven't forgotten them. Draw uncertainty bars by indicating the “best guess” value (typically either a single measured value or mean of a set of measurements) with a dot, and then drawing "bars" through the dot, whose lengths span the 95% confidence range of that value. An example of uncertainty bars is shown in Figure 4.3. The single data point plotted corresponds to a measured pendulum period of 1.93 s 0.03 s for an initial release angle of 20° 2°.
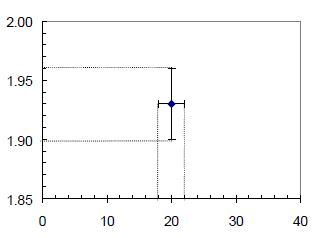
Figure 4.3: A graph of pendulum period T versus initial angle θ, showing how uncertainty bars indicate the uncertainty ranges associated with the displayed measurement value.
There are several other details that are important for polished graphs appearing in reports. You should make sure that each graph is labeled well. Use the following checklist to make a graph has the correct features and format.
◻ The graph has a figure number which you should refer to in the text of the report. ◻ The graph has an appropriate title which give more information than axis labels. ◻ The axes are clearly labeled, including units. ◻ The measured data points are clearly plotted, including uncertainty bars, but no lines connecting data points. ◻ If appropriate, a curve fit is included. The equation of the curve, the values of the best fit parameters, and the uncertainties of the parameters should also be given. ◻ If there is more than one data set on a graph, use different symbols and include a legend.
4.3 Making Graphs with Python
It is difficult to make graphs that follow all of the guidelines above by using Excel. In addition, Excel isn't very useful for fitting data with uncertainties. The matplotlib plotting library for Python (part of pylab) makes publication quality figures. Python can also be used for fitting data (see Ch. 5). On the computational tutorial webpage, there is extensive documentation on making graphs with Python. An example of making a scatter plot of data with uncertainty bars is shown below.
4.4 Graphical Analysis
Analyzing data on a graph often involves finding the slope and the intercept of a line. You will frequently manipulate your data so that the resulting graph is approximately a straight line (more about this in Ch. 6). The slope and/or intercept of such a line can usually be used to extract information about the physical system under investigation. Determining the slope and intercept of a linear graph is such a common and important task that you will learn to use a program to help you do it (see Ch. 5). However, it is good to be able to estimate roughly the slope and intercept of a rough graph by hand, so that you can see if your measurements are at least roughly correct before you enter them all into the computer. Estimating the slope and the intercept also allows you to check the computer's results, which will help you to catch simple errors like entering the data incorrectly.
Start by drawing by eye the line that you think best matches your data. This line doesn't need to pass directly through any data points, but should be the best fit to all of the data. Next, find the slope and the intercept of that line you've drawn. The slope of a line is defined as the “rise over run” or the change in the vertical coordinate value divided by the change in the horizontal coordinate value. To determine the slope, you must first choose two points on your line. They need not be actual data points but they must lie on your line. They should be about as far apart on the graph as possible to minimize the effects of estimating their locations. Mark each of those points by a dot with a circle around it to distinguish them from data points. Read the coordinates and of the points off the graph. Calculate the slope using
Using the slope, the -intercept is
Since the -intercept is defined as the value of where a line intersects the -axis (where ), you can also read the intercept directly off the graph as long as the graph shows the -axis.
4.5 Exercises
4.1 Make a polished graph of kinetic energy vs. distance fallen using the data provided in the table below. Use the checklist in section 4.2 to make sure that you have included everything.
Distance fallen (m) | Kinetic energy (J) |
---|---|
0.200 ± 0.003 | 2.00 ± 0.10 |
0.400 ± 0.003 | 3.78 ± 0.15 |
0.500 ± 0.003 | 5.65 ± 0.20 |
0.800 ± 0.003 | 8.01 ± 0.25 |
1.000 ± 0.003 | 9.82 ± 0.30 |
4.2 Draw what you think is the best possible line through the data points in the graph you just created in the previous exercise, and find the slope and intercept of this line.